Robin McLeod Lecture:
Using Big Data to
Solve Problems in
Transplantation
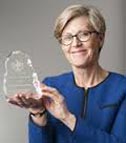
Robin McLeod
|
Robin McLeod is celebrated
by the lecture in
Surgical Epidemiology
given in her name each
year in the Department
of Surgery. The
Lecture honours her
distinguished career as
a surgeon, teacher and
clinical scientist. Robin
has over 350 publications
and has the highest
teaching effectiveness and academic citizenship
scores in the Department. Chairman Jim Rutka
described her at the introduction of the Lecture,
quoting from Marry Poppins - “she is practically
perfect in every way”.
|
Dorry Segev, this year’s McLeod Lecturer, is Associate
Vice - Chair of Surgery at Johns Hopkins. He is a transplant
surgeon and an internationally recognized expert in
organ allocation. Dorry has been instrumental in driving
transplant policy at the local, regional and national
level. He received his undergraduate degree in Computer
Sciences from Rice University in Houston and his medical
degree from Johns Hopkins. He completed general
surgery residency and an abdominal organ transplant fellowship
at Hopkins, where he also pursued and obtained
a PhD in biostatistics and clinical investigation. He has
been on the faculty since 2006, publishing more than
250 papers in leading journals, including the Journal of
American Medical Association, the New England Journal
of Medicine, and Lancet. He has received many prestigious
awards, including the Jacobson Promising Investigator
Award of the American College of Surgeons. His mentors
include Marta Zeiger, an endocrine surgeon at Hopkins,
paediatric surgeon Patricia K Donahoe at Massachusetts
General Hospital, and Robert A. Montgomery, Chief of
the Division of Transplantation at Johns Hopkins.
Dorry began by telling us that in the Big Data era
transplantation has a particularly useful dataset because
of the scarcity of organs and the long waiting lists. In
addition, there is an abundance of payer and pharmaceutical
claims data, giving hundreds of thousands to
millions of data points. “Big Data of this type can be
used very effectively through linkages. It is free of selection
bias, such as occurs when an individual service or
institution reports its experience. It is less granular than
institutional reports.”
Dorry has been successful in changing the law against
transplanting organs from patients with infectious diseases
(the IRD- infectious risk donors). There was a
federal law passed in 1984 against transplantation from
IRD patients. Dorry went to the U.S. Congress, taking
advantage of his proximity to Washington. He became
well versed in the ways of Washington, and especially
the effectiveness of knocking on doors, meeting intelligent
young staff personnel of Congressmen. By telling
them “how many people are affected by the problem,
how many lives can be saved, and what the cost will be
to benefit the constituents of the politicians who are
influential in getting the Bill approved and eventually
signed by the President.” He has had extraordinary success
in this quest. He told us how he used the National
Inpatient Sample, a large dataset, and found the number
of donors that could be used for transplantation if the
law were changed. He looked at an HIV dataset from
18 sites and linked it to the NIS (National Inpatient
Sample). $500,000 per patient could be saved if transplant
were substituted for dialysis. If the law against IRD
transplantation were changed, there would be an enormous
saving to the Medicare Program. On the basis of
Dorry’s data, President Obama signed the “Hope Act”,
reversing the 1984 law prohibiting IRD treatments.
|
Antibodies to the HLA antigens result in ineligibility
for about 20,000 potential kidney transplant recipients.
Dorry worked to resolve this problem by exchange
transplants. At the time that he started, organ exchange
(a swap among 2 pairs of incompatible individuals) was
illegal by reason of the laws against exchange of “money,
or anything of value” to purchase a transplant. A kidney
from another person was viewed by the Courts as a valuable
payment. Dorry approached this problem using the
tongue- in- cheek axiom of computer geeks. “If we don’t
have data, we make data”. This is shorthand for using
simulation to develop a convincing numerical argument.
There are between 1,500-3,000 incompatible pairs based
on anti HLA antibodies.
Working with Sommer Gentry, the mathematician
to whom he is married, Dorry and his colleagues got
the Charlie W. Norwood Living Organ Donation Act
passed and signed by President Bush. The Monte Carlo
simulation revealed that the loss ofdkidneys based on
high titres of antibody could be substantially reduced by
desensitization. Matching a desensitized patient with a
“counterfactual (i.e. a patient who has not been desensitized)
showed that there was a substantial improvement
in survival”. These data convinced Medicare to pay for
the desensitization process.
He then worked on the data supporting the clinical
maxim that black patients fared better on dialysis than
whites, so they were erroneously being less favoured for
transplantation. Nephrologists’ belief in this categorical
misconception was based on their experience with predominantly
older patients that they care for in dialysis
clinics, but the maxim was untrue for younger patients.
Using big data, Dorry was able to calculate the risk
of subsequent chronic kidney diseases in kidney donors.
This figure of 37 per 10,000 donors was derived using
social security data and National Health and Nutrition
Examination Survey (NHANES) data. “We made a
calculator from this data which will soon be published
in the New England Journal of Medicine. “When you
learn that there is a donor available, they are never perfect.
It’s like house hunting - there is always a problem
that has to be taken into consideration or modified”. For
example, the IRD donors (infectious risk donors) may
have a history of intravenous drug use or sex work. 20%
of potential donors are IRD. In the past, they have been
disqualified from transplantation, but with 100,000
patients on the waiting list for a kidney and 50% mortality
of waiting patients, why not use IRD donors? For
this problem Dorry used the Markov decision process
model. This mathematical empirical study revealed a
10% difference in outcome between IRD and no-IRD
donations. This difference, though significant, is still
well-worth the risk to many patients facing a 50% risk
of death on the waiting list.
In summary, Dorry’s big data studies helped him to
re-write laws, to gain funding for the desensitization process,
to clarify clinical misconceptions, to develop a donor
risk calculator, and to introduce the use of infectious risk
donors to help resolve the transplant organ shortage.
David Urbach asked about contrasts between Canada
and America in terms of the Big Data studies. Dorry
answered that the problems that he has been addressing
are seen throughout the world, but we can model
selection on regional data, including questions like “Is it
reasonable to put kidneys from older patients into young
patients? Simulators can help, but modelling is extremely
helpful for policy decisions like this. John Marshall asked
whether this very efficient use of available data could
be helpful to less developed countries like Bangladesh.
Dorry answered that calculators of the type he has developed
are now trusted, they no longer meet the “garbage
in, garbage out bias” that characterized an earlier age.
The spread of the electronic medical record is not limited
to more developed countries. Jim Rutka asked how
Dorry managed to get a Bill to reverse a standing law
“rocketed through the Congress, when we hear so much
about gridlock in the US capital”. “Knocking on doors
and persistence was the secret of success, but was not
exactly rocket speed, as the paper was published in 2010,
and news media picked the information up in 2011 and
the Bill was signed in 2013.”
In closing, David Urbach reminded us that Robin
McLeod had blazed the trail to changing practice
through epidemiological studies, brilliantly exemplified
by Dorry’s work.
M.M.
|